CLunch
CLunch is the weekly Computational Linguistics lunch run by the NLP group. We invite external and internal speakers to come and present their research on natural language processing, computational linguistics, and machine learning.
Interested in attending CLunch? Sign up for our mailing list here.
View older talks at the CLunch archive.
Past Talks
Past talks from the current and previous semesters are shown below. View older talks at the CLunch archive.
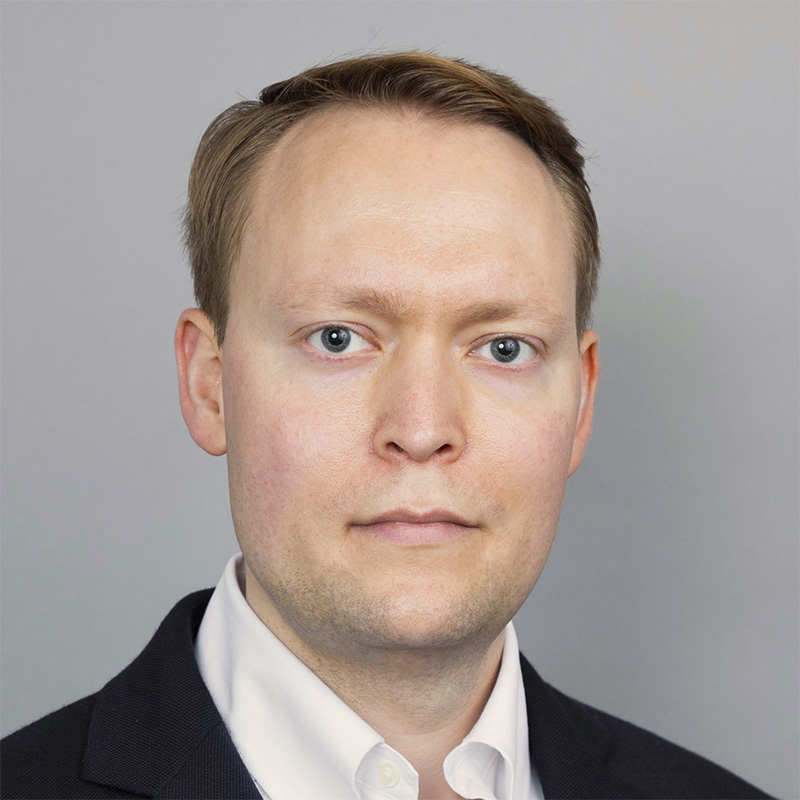
University of Southern California
May 05, 2025
Embracing Language as Grounded Communication
Language is not text data, it is a human medium for communication. The larger part of the natural language processing (NLP) community has doubled down on treating digital text as a sufficient approximation of language, scaling datasets and corresponding models to fit that text. I have argued that experience in the world grounds language, tying it to objects, actions, and concepts. In fact, I believe that language carries meaning only when considered alongside that world, and that the zeitgeist in NLP research currently misses the mark on truly interesting questions at the intersection of human language and machine computation. In this talk, I’ll highlight some of the ways my lab enables agents and robots to better understand and respond to human communication by considering the grounded context in which that communication occurs, including neurosymbolic multimodal reasoning, natural language dialogue and interaction for lifelong learning, and utilizing NLP technologies on non-text communication.
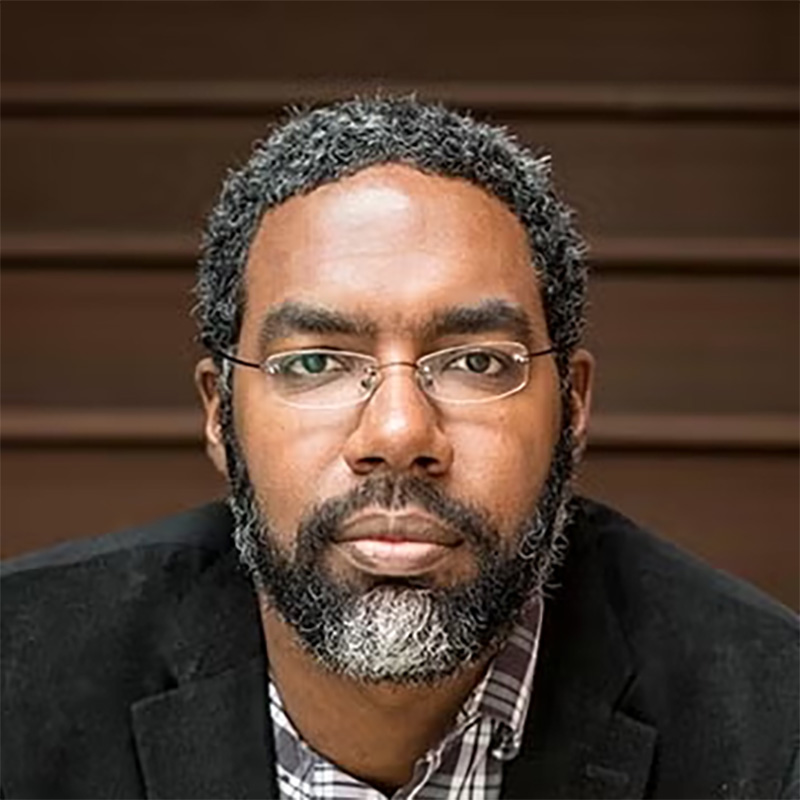
University of Pennsylvania
April 28, 2025
The Post-API Age: Past, Present, and Future
NLP and machine learning research has long relied on readily available, high-quality data from large social media platforms, but obtaining such data has not always been easy. In a brief 2018 essay titled “Computational Research in the Post-API Age” (published in the journal Political Communication), I warned researchers that the days of free and easily accessible digital communication data were coming to an end. The essay was initially inspired by the prohibition of automated data collection from Facebook’s Graph API, which occurred in March 2018 in the wake of the Cambridge Analytica scandal. This corporate decision effectively eliminated most authorized means of collecting Facebook data (except those in direct collaboration with Meta researchers) until the Crowdtangle service was opened to academic researchers in the summer of 2020. Meta shelved Crowdtangle in August 2024, replacing it with yet another data access regime, the Meta Content Library. Other social platforms, including Twitter/X, Reddit, and TikTok, have also substantially modified their data access policies over the years.
Some of the issues I raised in my Political Communication essay are still relevant today, while others are less so. This presentation, which is based on an invited journal article, will build on my earlier piece in three ways: first, it will recount a concise history of social media data access informed by official documentation and my own professional observations as one of the first Communication researchers to analyze social media data computationally. Second, it will sketch the present state of digital communication data access in context with past such “ages,” making practical recommendations and generally characterizing the moment for posterity. The third section will be devoted to the future, but instead of making predictions, it will adopt a normative approach, advocating for corporate and governmental data access policies that balance the researcher’s interest in data usability, the public’s interests in privacy and impactful research, and business interests in transparency and good corporate citizenship.

Johns Hopkins University
April 21, 2025
AI for Science and Science of AI
AI has seen remarkable advancements, driven largely by the increasing capabilities of LLMs and have sparked excitement around their transformative potential across applications—particularly their role in accelerating scientific discovery.
In the first part of this talk, I will focus on our work in AI for science, emphasizing the importance of addressing "aggregative questions" in scientific inquiry. While traditional scientific questions often are “information seeking” queries, (looking for specific information from individual papers), many critical scientific challenges require synthesizing abstractions of evidence across diverse sources. I will highlight our approaches to enabling LLMs to effectively tackle such aggregative tasks.
In the second part, I will turn to the science of AI, exploring foundational challenges in training and deploying LLMs. This includes issues related to data quality (such as temporal degradation of knowledge after the model’s training cutoff) and the difficulties posed by imbalanced datasets during pretraining. Our work aims to better understand and address these limitations to improve the reliability of LLMs in scientific contexts.
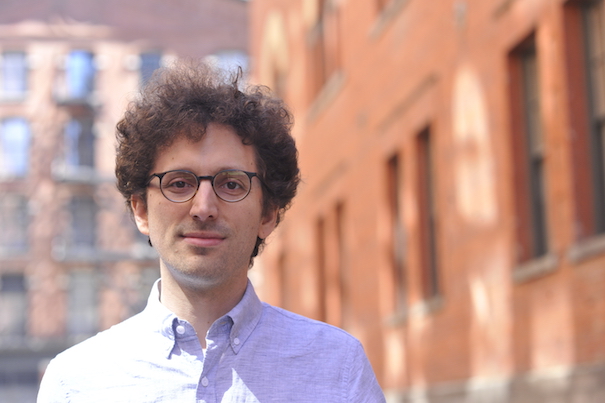
Cornell University
April 14, 2025
Post-training via Interaction
Post-training language models is key to exposing and refining their abilities. This process often relies on costly, annotated data, even when RL is used. However, deployment interactions are rich with learning signals, and hold the potential for on-policy, low-cost post-training. I will describe three projects that demonstrate this, and show under-utilized learning behaviors: (a) LLMs can inspect their own interactions to continually improve, with no annotation effort; (b) jointly reasoning about comprehension and generation of language in LLMs dramatically improves learning and reduces sample complexity; and (c) LLMs have the ability for RL in context, thereby learning from rewards with no parameter updates. Across the three projects, we show robust behaviors over many interactions, often through live deployments. Taken together, our work shows the rich learning landscape model interactions expose.
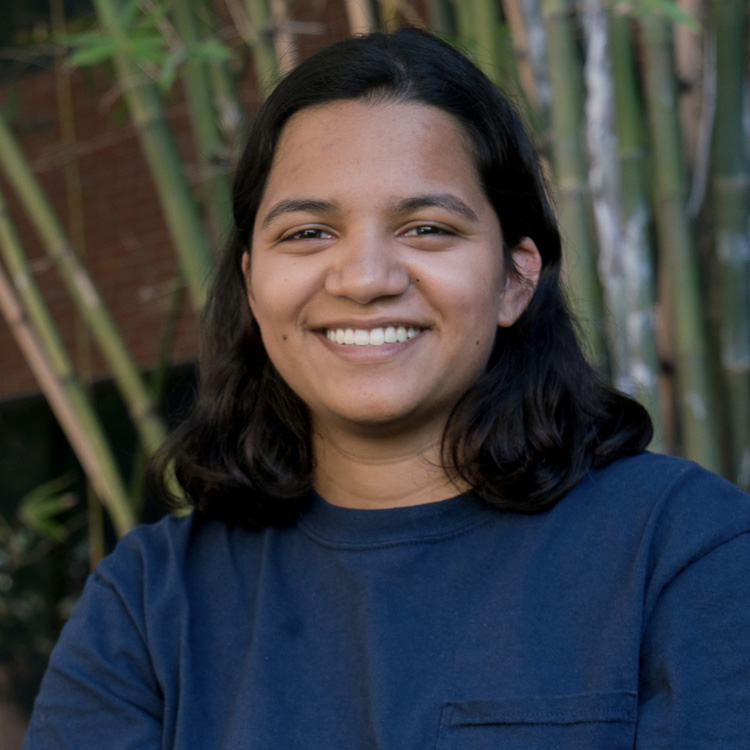
Carnegie Mellon University
April 07, 2025
Towards Artificial Social Intelligence: Grounded Social Reasoning in Multimodal Models
Advancing social intelligence in AI systems is important for these systems to effectively navigate human social interactions and work with and around humans. This talk will focus on grounded social reasoning abilities of multimodal models. I will discuss Social Genome, a modeling challenge that tests the abilities of multimodal models to reason about human social interactions, by interpreting and integrating embodied multimodal communicative behaviors and external social knowledge. I will also discuss core technical challenges and opportunities to advance artificial social intelligence, with perspectives anchored in social intelligence concepts and prior research from several computing communities.
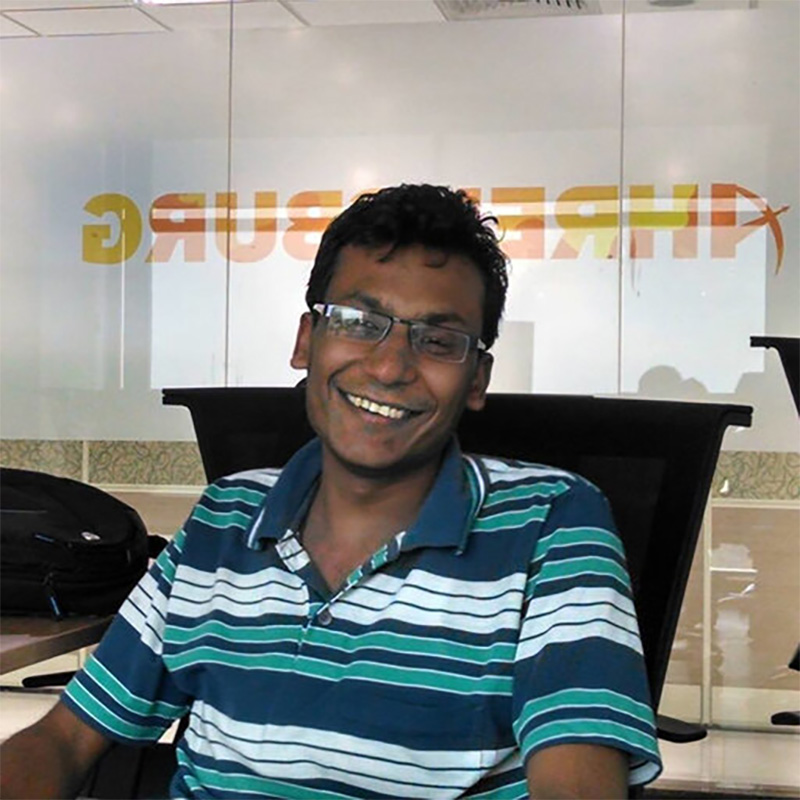
Arizona State University
March 31, 2025
Reasoning for Multimodal Semi-structured Tables
In this talk, I'll walk through some of the latest AI advancements that address the challenges of working with complex data, with a focus on improving reasoning for both tabular and multimodal data.
I'll begin by introducing H-STAR, a hybrid algorithm that combines symbolic and semantic reasoning to enhance question answering for tabular data. By leveraging multi-view table extraction and adaptive reasoning, H-STAR has shown great potential in improving reasoning across tabular datasets.
Then, I'll introduce MMTabQA, a dataset we developed to evaluate how AI systems manage multimodal tables that integrate structured text and images. Our research reveals where current models struggle to process these diverse data types, highlighting key areas for improvement.
To wrap up, I'll discuss open challenges and future directions, including expanding reasoning capabilities to other complex data types—such as charts, maps, and flowcharts—and enhancing AI systems' robustness in handling numerical, temporal, and visual reasoning, particularly with large (vision) language models.
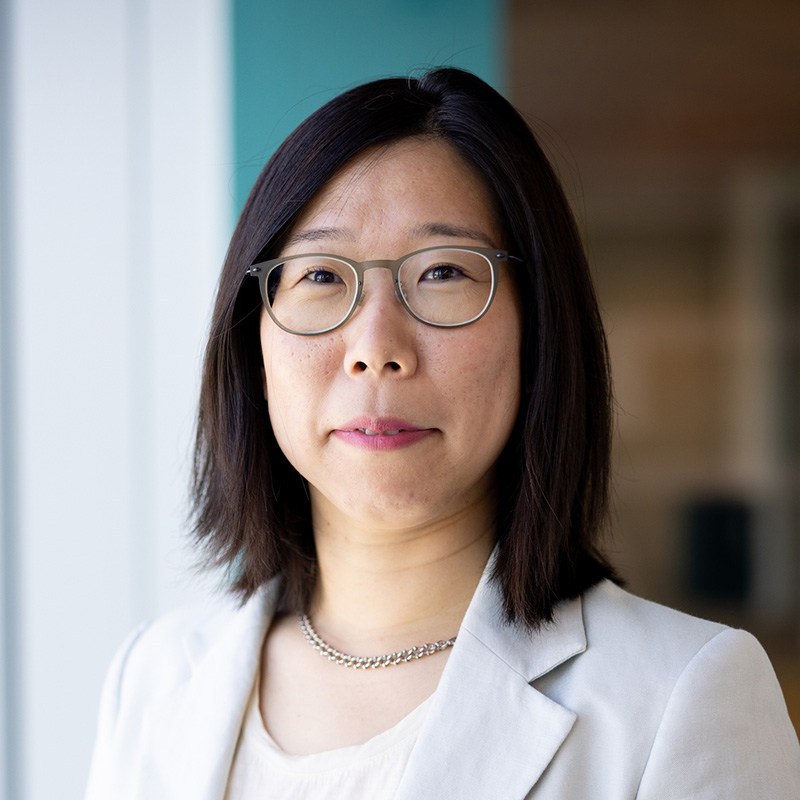
Georgia Institute of Technology
March 24, 2025
Cultural Bias and Privacy Protection in Large Language Models
In this talk, I will explore two key aspects of large language models (LLMs) and their pre-training data: cultural bias and privacy preservation. First, I will present a systematic study on LLMs' favoritism toward Western culture. Our approach involves curating a dataset of over 20,000 cultural items, including food, clothing, individuals, religious sites, and more, to contrast Arabic and Western cultures. We assess cultural biases across multilingual LLMs (e.g., GPT-4, Aya) through natural prompts, story generation, sentiment analysis, and named entity recognition (NER) tasks. A key finding suggests heavy reliance on Wikipedia data during pre-training may have contributed to the bias toward Western concepts in non-Western languages.
Next, I will showcase PrivacyMirror, an LLM-powered tool designed to help users safeguard their personal information. Leveraging insights from an HCI study with real Reddit users, PrivacyMirror reveals challenges and opportunities which shape ongoing efforts to enhance user-centric privacy protection.
To conclude, I will briefly discuss recent research on the temporal robustness of LLMs, particularly their ability to handle neologisms, and advances in human-AI interactive evaluation.
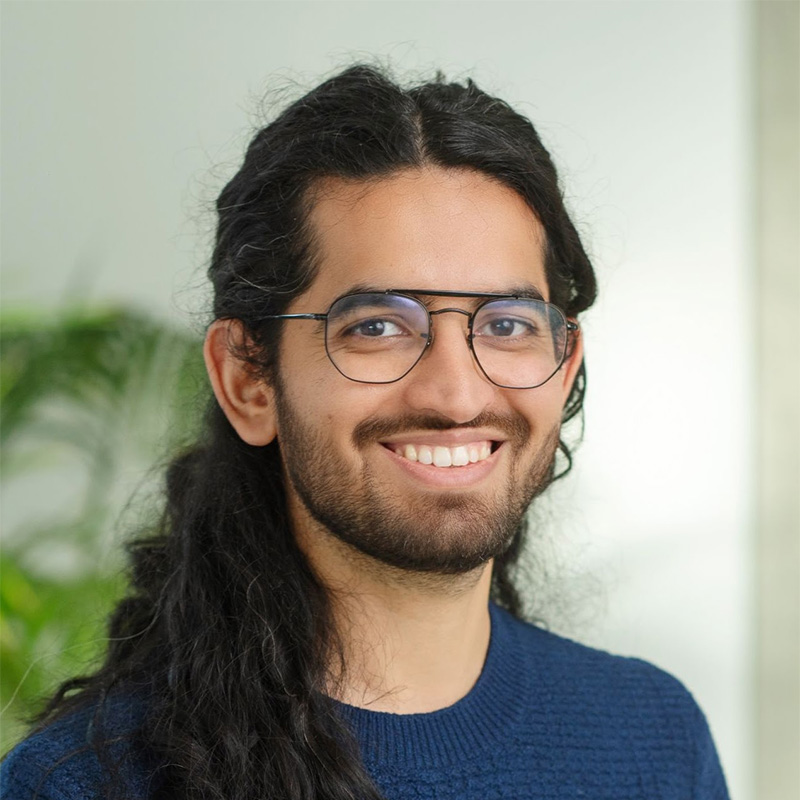
The Ohio State University
March 17, 2025
Towards Continually Adaptable Multilingual Language Models
Subword tokenization in multilingual models presents significant challenges, particularly for non-Latin scripts and low-resource languages. Existing methods not only perform worse in these settings but also lead to higher costs in LLM APIs due to inefficiencies in token representation. Furthermore, incorporating emerging languages into pre-trained models remains difficult, with prior work often relying on heuristics like vocabulary expansion. To address these issues, I will first present MAGNET, a multilingual adaptive gradient-based tokenization algorithm that is jointly trained with a language model. Unlike standard tokenization approaches, MAGNET operates at the byte level and learns to predict segment boundaries dynamically via a tokenizer sub-module. A simple hyperparameter allows controlling over segmentation granularity, ensuring equitable treatment across languages. Its end-to-end training enables seamless adaptation of language models when fine-tuned on new languages. In the second part, I will introduce ongoing work on continually adapting multilingual language models to new languages. This work investigates how effectively models can be extended to languages with varying levels of resources and linguistic relatedness while preserving performance on previously supported languages. We study the trade-offs between adaptation efficiency, catastrophic forgetting, and cross-lingual generalization, providing insights into practical strategies for real-world multilingual NLP.
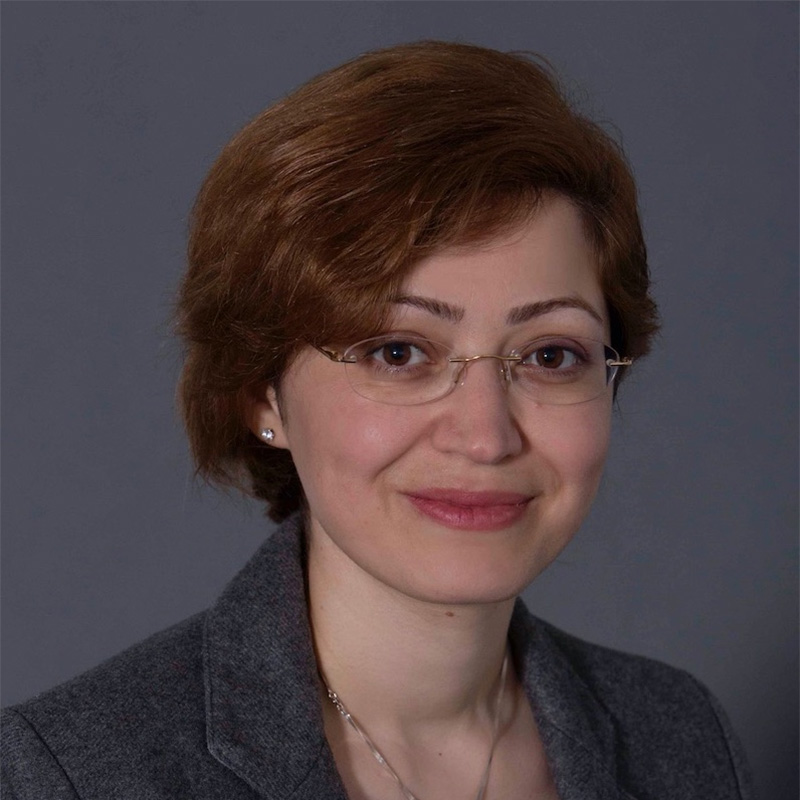
Michigan State University
March 03, 2025
Compositional Reasoning for Natural Language Comprehension and Grounding Leveraging Neuro-Symbolic AI
Recent research indicates that large language models lack consistent reliability in tasks requiring complex reasoning. While they may impress us with fluently written articles prompted by user input, they can easily disappoint by displaying shortcomings in basic reasoning skills, such as the functional understanding of 'left is the opposite of right', let alone grounding such concepts in diverse real-world situations involving perception and action. To address real-world problems, computational models often need to involve multiple interdependent learners, along with significant levels of composition and reasoning. In this talk, I will present our findings regarding reasoning challenges of LLMs and discuss how symbolic representations can leverage the capacity of neural models for compositional reasoning over complex linguistic structures, grounding language in visual perception, combining multiple modalities of information and handling uncertainty. I will highlight our efforts in Neurosymbolic modeling and introduce DomiKnowS, our developed library that facilitates such modeling. The DomiKnowS framework exploits both symbolic and sub-symbolic representations to solve complex, AI-complete problems and seamlessly integrates symbolic and logical knowledge into deep models through various underlying algorithms.

Dataminr
February 24, 2025
A Brief History of Natural Language Processing
The title says it all! As the field of Natural Language Processing (NLP) continues to make incredible strides and advancements, it's important to take a step back and use the past to understand the current transformations. Drawing from literature and interviews, we'll dive into the early years of NLP and explore some of the major themes, trends, and personalities that paved the way for the cutting-edge technology we have today.
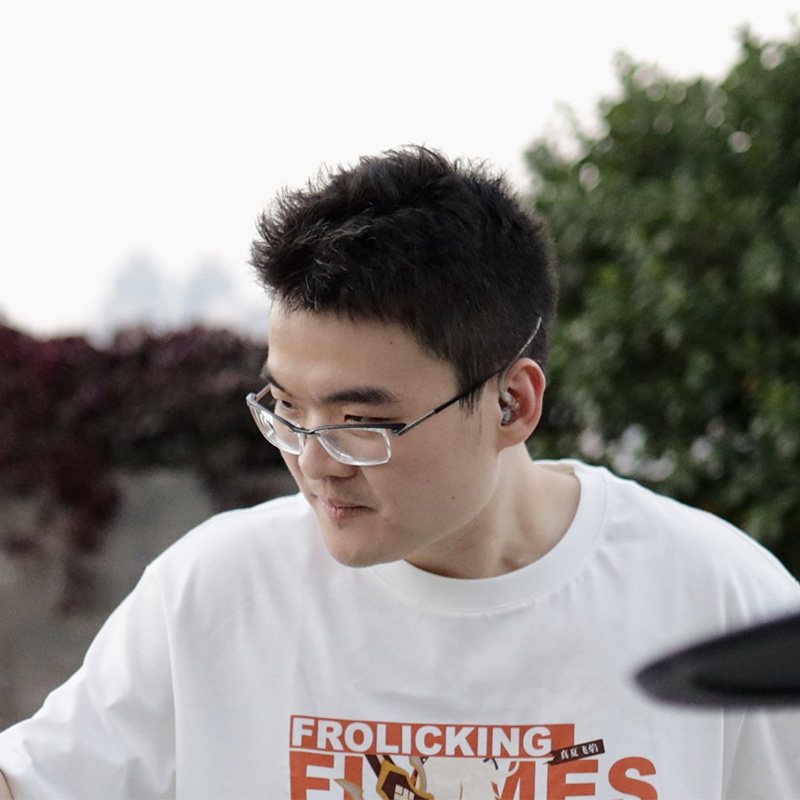
Drexel University
February 17, 2025
Executable and Trustworthy Planning with Large Language Models
While large language models (LLM) can provide decent instructions, they are far from able to come up an executable and trustworthy plan for a particular user or agent, grounding to their specific situation and needs. To address this, I advocate for the methodology of using LLM as a code generator to create a formal representation of the planning environment. In conjunction with tools in classical AI planning, a plan can be found deterministically and faithfully. In this talk, I will discuss two strands of efforts. The first tackles fully-observed planning domains, where the model is given complete information and must propose a complete plan that satisfies given constraints. The second tackles partially-observed planning domains, where the model makes partial observations about the environment, propose partial plans, and iteratively acquire knowledge to complete a task. In both settings, we show that state-of-the-art models like DeepSeek-R1 and gpt-4o are heavily challenged by even the simplest tasks like rearranging or looking for objects. When prompted to generate the planning domain definition language (PDDL) input into a solver, LLMs outperform generating the plans directly. Even so, both syntactic and semantic errors point to LLMs' weakened ability to generate formal representations, especially when the language or domain is underrepresented in their pre-training.
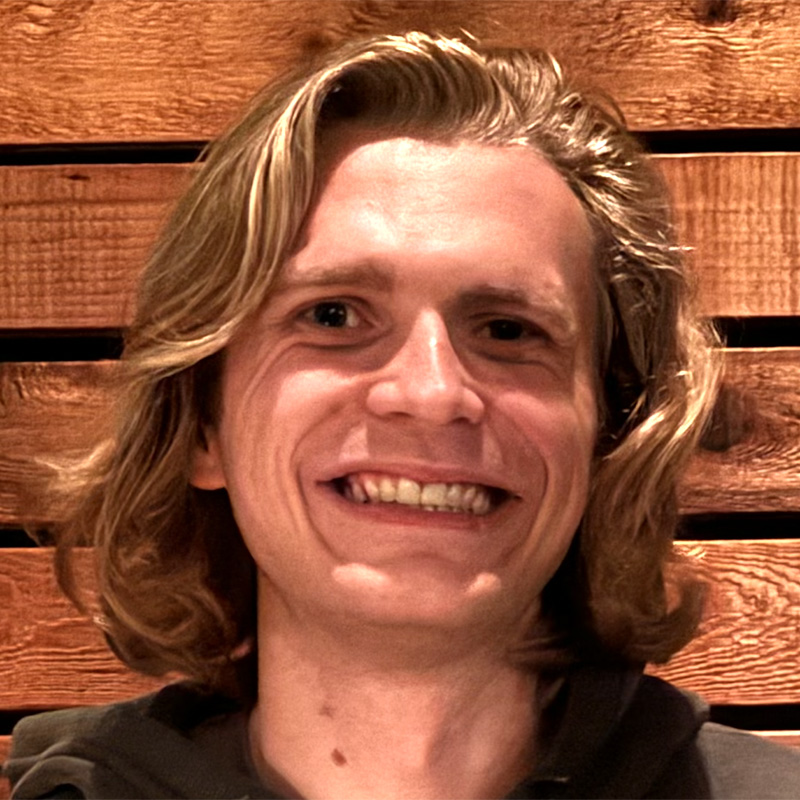
University of Washington
February 10, 2025
Pluralistic Alignment: A Roadmap, Recent Work, and Open Problems
Much alignment work assumes that there is a single set of human preferences or values that AI systems should align to. Pluralistic alignment, on the other hand, is concerned with integrating diverse human values and perspectives into alignment algorithms and evaluations. In this talk, I will start by presenting our roadmap to pluralistic alignment, offering definitions and scaffolding for research in the area. I will present one proposed algorithmic framework to support pluralism through modular specialist systems, along with a dataset and system to improve computational value-modeling. I will conclude with future research directions and open questions in the area.
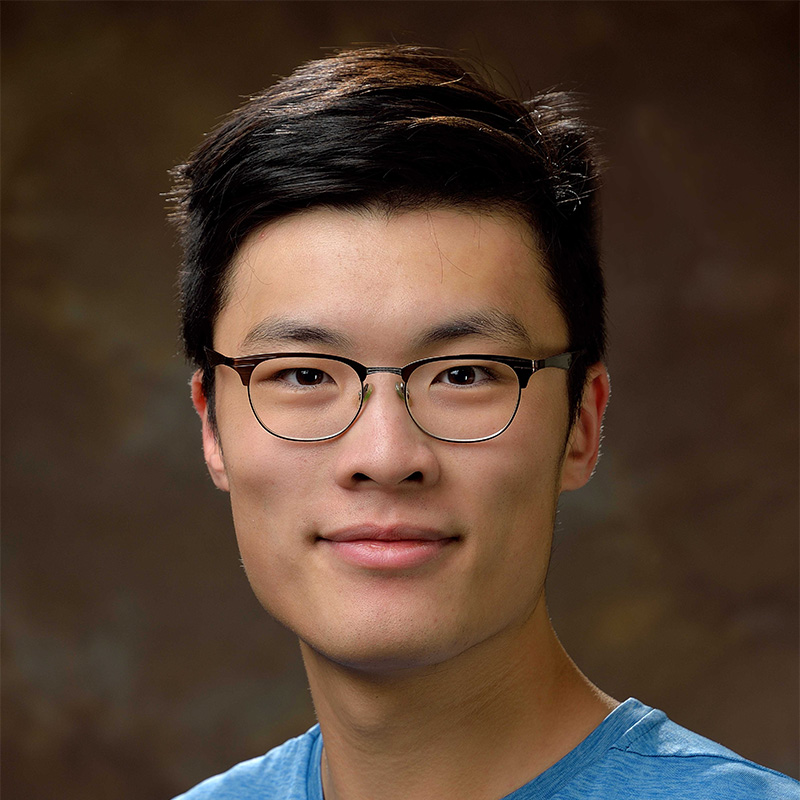
Arizona State University
February 03, 2025
Enforcing Abstraction with Artificial Bottlenecks for Complex Machine Reasoning
Human reasoning comes from trial and error on an abstract level: we learn from past experiences, conceptualize atomic operations and notions, and recompose them in new situations and scenarios. Such abstraction and conceptualization are the key sources of generalization. On the other hand, recent successes in machine reasoning are primarily from end-to-end training (e.g., next-word prediction and task-specific supervision), where models tend to rely more on memorization than generalization. Such observations motivate us to encourage models to perform high-level abstractions when learning. In this talk, I will discuss my recent works and methods that force model abstractions with artificial bottlenecks, where we deliberately remove essential information from the reasoning process so that models have to reason abstractively, similar to how computer programs conceptualize input values with variables and operations. These works show promising future research directions of model generalization and trustworthiness.
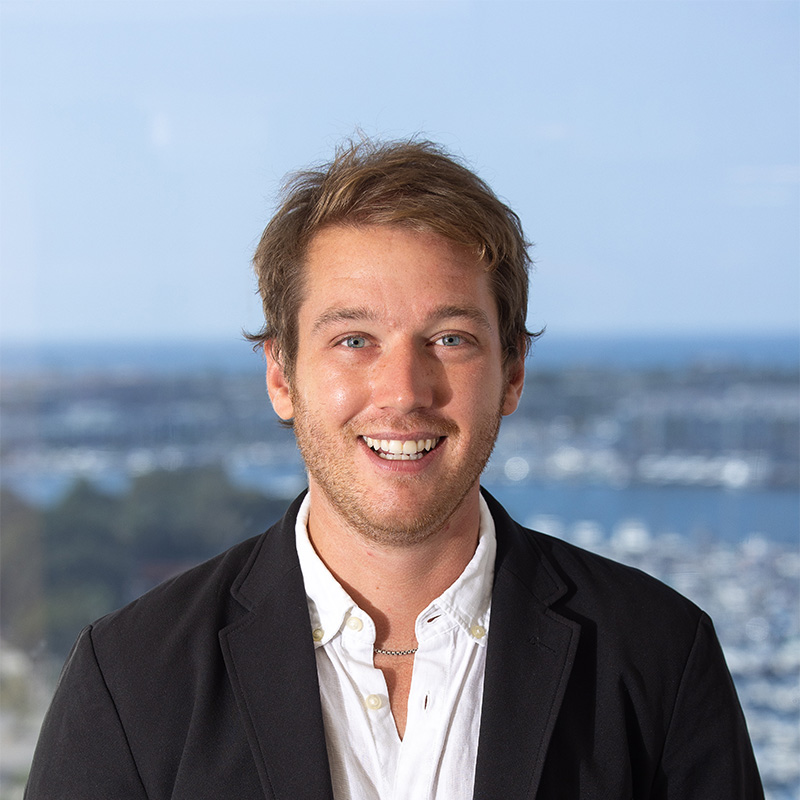
University of Southern California
January 27, 2025
Planning in Creative Contexts
Recent modeling innovations incorporate planning — or reasoning about actions and future states, exhibited by models like GPT-o1 and Deepseek's R1 — and have demonstrated impressive performance gains in areas like mathematical problem-solving and computer coding. However, such domains are characterized by well-defined goals (or rewards); for many human-centered creative tasks, rewards are not as clearly defined and it is thus not clear how current planning approaches can transfer. In this talk, I will outline a research agenda that can enable us to make progress in these areas. I focus on tasks related to journalism, with specific focus on retrieving a set of sources relevant to a news story. I will show how we can make inferences about human actions based on limited state-observations (a process known to cognitive psychologists as "emulation", but as yet unexplored in machine learning) and how these inferences can help us learn human values and rewards.
Carnegie Mellon University
December 09, 2024
Environmentally Sustainable AI: Challenges and Solutions
Modern machine learning powered by deep learning and large language models has the potential to help humans tackle big challenges such as climate change. At the same time, training and deploying these models comes at an increasingly high computational cost, with corresponding costs to the environment. The relationship between ML and climate change is complex, with great potential for ML to have either positive or negative impacts on the environment. In this talk I’ll discuss what we know about the environmental impact of LLMs and ML more broadly, where there are currently gaps in our knowledge, and what we can do to shape a future where the benefits of AI outweigh the costs.
University of Toronto
December 02, 2024
Progress on a permissively licensed text dataset
Large language models (LLMs) are typically pre-trained on huge quantities of copyrighted text. In the face of raising concerns and lawsuits from rights holders, companies developing LLMs claim that training on copyrighted data is fair use (which can and will only be settled in court), and in any case, further claim it would be “... it would be impossible to train today’s leading AI models without using copyrighted materials.” However, this claim has not been sufficiently tested. I will present our ongoing work (in collaboration with EleutherAI, AI2, and pleIAs) on developing a large collection of permissively licensed text data, amounting to many terabytes of text from an unusually diverse range of more than 25 sources, including governmental texts, historical books, research papers, educational video transcripts, and more. If the pieces fall into place in time, I'll also be able to present some preliminary evaluations of a language model trained on our dataset.
University of Michigan
November 25, 2024
Detecting implicitly harmful language in political discourse
When discussing politics, people often use subtle linguistic strategies to influence how their audience thinks about issues, which can then impact public opinion and policy. For example, anti-immigration activists may frame immigration as a threat to native born citizens’ jobs, describe immigrants with dehumanizing vermin-related metaphors, or even use coded expressions to covertly connect immigration with antisemitic conspiracy theories. This talk will focus on my recent and ongoing work in developing computational approaches to analyze (1) dogwhistle communication and (2) the framing of immigration via dehumanizing metaphors. I will discuss how I draw from multiple social science disciplines to develop typologies and curate data resources, as well as how I build and evaluate natural language processing models for detecting these strategies. I further analyze the use of these strategies in political discourse across several domains, and assess the implications of such nuanced rhetoric for both society and technology.
Allen Institute for AI
November 11, 2024
OLMo: Accelerating the Science of Open Language Models
Recently, we have seen tremendous progress in the field of language models (LMs), with the release of numerous open models and closed API systems. However, fewer and fewer disclose how they are created: Which corpora do they use? How are they trained? How much energy do they consume? In this talk, I will provide an overview of OLMo (https://allenai.org/olmo), an initiative at Ai2 aimed at creating transparent artifacts and tools that advance the science of LMs. I will discuss current and upcoming releases, such as Tulu, Dolma, OLMo, OLMoE, as well as the goals and ethical/legal considerations of this initiative.
University of Pennsylvania
November 04, 2024
A More Natural and Complex Question Answering Benchmark
An important and highly useful application of large language models is answering information-seeking questions. Ideally, an evaluation benchmark for this task should include natural questions that reflect real-world users' goals. However, existing QA benchmarks contain questions that are either natural but simple (answer lies in a single sentence) or complex questions that are machine generated and are often contrived. To address this gap we introduce MONACO, a new benchmark of More Natural and Complex QA. The questions in MONACO are all manually written and express a diverse set of user goals. In terms of complexity, MONACO questions require aggregating information from 34 documents on average -- more than double that of previous list QA tasks. Overall, we collected over 1,800 multi-step, 8,000 list and 110,000 single step natural questions complete with answers and document attributions. We use MONACO to benchmark the performance of the top performing LLMs and explore the strengths and pitfalls of popular prompting techniques like chain-of-thought and decomposed prompting.
Carnegie Mellon University
October 28, 2024
Towards a science of human-AI teams
AI models have the potential to support and complement human decision-makers and users. And yet, the deployment of human-AI teams still faces practical challenges. I’m interested in developing a more principled workflow for building human-AI teams. In particular, this talk will focus on answering two questions: (1) what the right evaluation paradigms are to measure team performance and (2) what are interaction mechanisms that can facilitate the appropriate usage of AI support. I will discuss how existing ML/NLP literature has attempted to answer each of these questions, their limitations, and promising alternatives.
Cornell University
October 21, 2024
Collecting and Learning from Human Feedback
Human Feedback has emerged as an important ingredient to align large language models. However, it is challenging to both collect high quality human feedback, and ensure that reward models trained on this feedback capture the right axes of preferences. In this talk, I will present our work on developing a robust pipeline for data collection that improves agreement. I will further describe a thrust of our work that investigates a peculiar phenomenon that occurs when training language models with human feedback: model outputs are substantially longer. Our work shows that performance improvements after RLHF are largely due to increased length, instead of other important features. We test a comprehensive set of length-countering interventions, and identify reward models as the dominance source of this bias.
Stanford University
October 14, 2024
Instruction Following without Instruction Tuning
This talk is about a few curious results on what makes language models follow instructions. When we want a language model to follow instructions, we often finetune it on instruction-response pairs and hope it generalizes -- this is (explicit) instruction tuning. I'll discuss _implicit instruction tuning_ -- we discovered that sometimes, when we finetune a language model on an objective that seems deficient compared to instruction tuning, the resulting finetuned model follows instructions anyway. This happens when we train a model to predict responses without conditioning on instructions (response tuning), and when we train only on a single task (like generating only poetry, or only recipes.) To dig into why implicit instruction tuning seems so common, I'll make concrete how simple it is to make a language model follow instructions by showing how to with a rule-based helper. As a takeaway: when you adapt a language model for a specific task, it's possible that it might (surprisingly?) act as a general-purpose chatbot for highly dissimilar inputs.
University of Washington
October 07, 2024
The Enigma of LLMs: on Creativity, Compositionality, and Paradoxes
That we can create LLMs doesn’t mean that we know LLMs. In this talk, I will discuss the puzzling questions and paradoxes we face with LLMs, from creativity to compositionality.
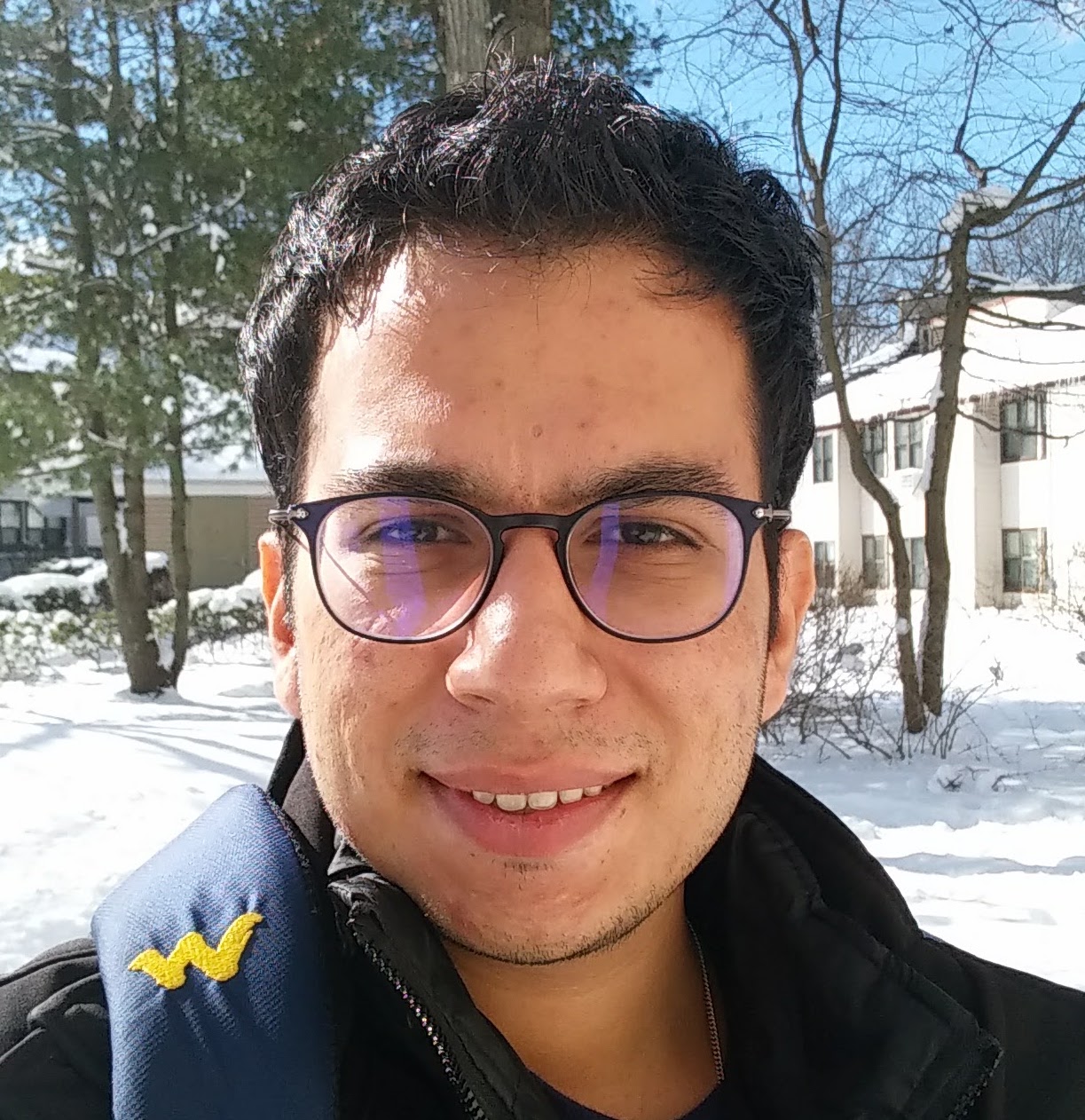
Stony Brook University
September 30, 2024
AppWorld: Reliable Evaluation of Interactive Agents in a World of Apps and People
We envision a world where AI agents (assistants) are widely used for complex tasks in our digital and physical worlds and are broadly integrated into our society. To move towards such a future, we need an environment for a robust evaluation of agents' capability, reliability, and trustworthiness. In this talk, I’ll introduce AppWorld, which is a step towards this goal in the context of day-to-day digital tasks. AppWorld is a high-fidelity simulated world of people and their digital activities on nine apps like Amazon, Gmail, and Venmo. On top of this fully controllable world, we build a benchmark of complex day-to-day tasks such as splitting Venmo bills with roommates, which agents have to solve via interactive coding and API calls. One of the fundamental challenges with complex tasks lies in accounting for different ways in which the tasks can be completed. I will describe how we address this challenge using a reliable and programmatic evaluation framework. Our benchmarking evaluations show that even the best LLMs, like GPT-4o, can only solve ~30% of such tasks, highlighting the challenging nature of the AppWorld benchmark. I will conclude by laying out exciting future research that can be conducted on the foundation of AppWorld, such as benchmarks and playground for developing multimodal, collaborative, safe, socially intelligent, resourceful, and fail-tolerant agents.
Johns Hopkins University Human Language Technology Center of Excellence (HLTCOE)
September 23, 2024
Takeaways from the SCALE 2024 Workshop on Video-based Event Retrieval
Information dissemination for current events has traditionally consisted of professionally collected and produced materials, leading to large collections of well-written news articles and high-quality videos. As a result, most prior work in event analysis and retrieval has focused on leveraging this traditional news content, particularly in English. However, much of the event-centric content today is generated by non-professionals, such as on-the-scene witnesses to events who hastily capture videos and upload them to the internet without further editing; these are challenging to find due to quality variance, as well as a lack of text or speech overlays providing clear descriptions of what is occurring. To address this gap, SCALE 2024, a 10-week research workshop hosted at the Human Language Technology Center of Excellence (HLTCOE), focused on multilingual event-centric video retrieval, or the task of finding relevant videos about specific current events. Around 50 researchers and students participated in this workshop and were split up into five sub-teams. The Infrastructure team focused on developing MultiVENT 2.0, a challenging video retrieval dataset consisting of 20x more videos than prior work and targeted queries about specific world events across six languages. Other teams worked on improving models from specific modalities, specifically Vision, Optical Character Recognition (OCR), Audio, and Text. Overall, we came away with three primary findings: extracting specific text from a video allows us to take better advantage of powerful methods from the text information retrieval community; LLM summarization of initial text outputs from videos is helpful, especially for noisy text coming from OCR; and no one modality is sufficient, with fusing outputs from all modalities resulting in significantly higher performance.
University of Pennsylvania
September 16, 2024
DataDreamer: Synthetic Data Generation and Reproducible LLM Workflows
Large language models (LLMs) have become an essential tool for NLP researchers in a wide range of tasks. Many now rely on LLMs for synthetic data generation, task evaluation, fine-tuning, and other model-in-the-loop workflows. However, challenges arise due to their scale, closed-source nature, and the lack of standardized tooling, which can hinder open science and reproducibility. In this talk, we present DataDreamer, an open-source Python library designed to help researchers implement LLM workflows more easily. DataDreamer also promotes best practices that support open science and improve reproducibility in research.
University of Pennsylvania
September 16, 2024
Advancing Multimodal AI: Integrating Modalities, Tackling Complex Challenges, and Enhancing Interpretability
Advancing AI systems that understand and integrate multiple modalities—such as images, language, audio, video, and 3D—has significant implications for real-world applications. A major challenge lies in developing AI models that can efficiently process diverse modalities while providing transparent and interpretable decision-making. This talk will highlight recent contributions, including X-InstructBLIP, a framework for aligning multimodal representations with language models for cross-modal reasoning; studies on bistable images that reveal how AI models interpret visual ambiguity; and ongoing work on visual unit testing to ensure robust, and interpretable multimodal reasoning.
University of Pennsylvania
September 16, 2024
Better Evaluations for Multimodal Generative Models
Multimodal generative models such as GPT-4o and DALL-E 3 are being developed at a rapid pace. While these models have incredible new abilities, we still mostly follow the same old paradigms when it comes to evaluating the language or images that these models produce. Consequently, these models' potential is constrained by outdated evaluation criteria. In this talk, we will introduce two new benchmarks: (1) BLINK, designed to assess core visual perception abilities that are not addressed by existing benchmarks for multimodal large language models; and (2) Commonsense-T2I, which tests whether the generated images align with real-life commonsense. Our findings show that current multimodal generative models perform significantly worse than humans on both benchmarks, highlighting potential pathways for future improvements.
Arizona State University
September 9, 2024
Can LLMs Reason and Plan?
Large Language Models (LLMs) are on track to reverse what seemed like an inexorable shift of AI from explicit to tacit knowledge tasks. Trained as they are on everything ever written on the web, LLMs exhibit “approximate omniscience”–they can provide answers to all sorts of queries, but with nary a guarantee. This could herald a new era for knowledge-based AI systems–with LLMs taking the role of (blowhard?) experts. But first, we have to stop confusing the impressive style/form of the generated knowledge for correct/factual content, and resist the temptation to ascribe reasoning, planning, self-critiquing etc. powers to approximate retrieval by these n-gram models on steroids. We have to focus instead on LLM-Modulo techniques that complement the unfettered idea generation of LLMs with careful vetting by model-based verifiers (the models underlying which themselves can be teased out from LLMs in semi-automated fashion). In this talk, I will reify this vision and attendant caveats in the context of our ongoing work on understanding the role of LLMs in planning tasks.